AI is one of the most critical factors in our lives today. It influences choices about loans, hiring, health care and even criminal justice. However, the bias in AI is a big issue. As the use of AI systems becomes more pervasive, it is essential to implement fair and ethical practices.
This guide covers how bias affects AI, how it affects the world around us, and how we can improve to create fairer AI systems.
Key Benefits: Why AI Fairness Matters
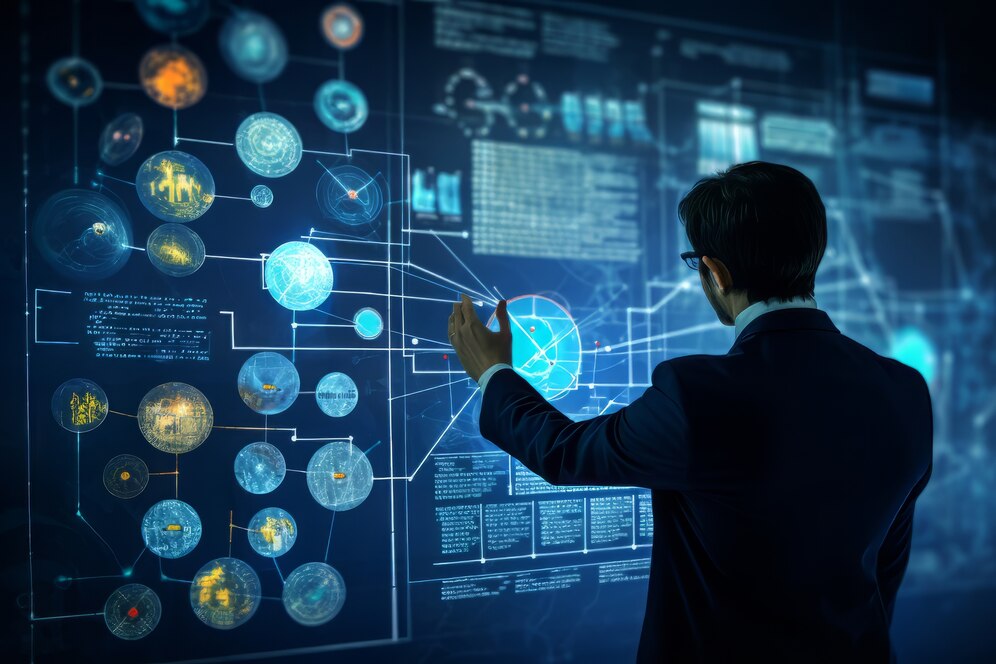
1. The Importance of Addressing AI Bias
AI systems greatly influence lives. Bias can lead to discrimination and reinforce societal inequalities. For example:
- Criminal Justice: Risk assessment tools can unfairly impact certain racial groups, resulting in unjust sentencing.
- Hiring Algorithms: Biased recruitment tools may favour specific demographics, reducing workforce diversity.
- Healthcare AI: Medical tools can be less effective for underrepresented groups, causing disparities in diagnosis and treatment.
Understanding algorithmic ethics is vital for ensuring AI serves all communities reasonably.
2. Real-Life Applications and Data-Backed Insights
AI bias has led to serious issues. Some notable cases include:
- COMPAS Algorithm: This tool in the U.S. criminal justice system inaccurately predicts recidivism, disproportionately affecting African American defendants.
- Facial Recognition Errors: MIT Media Lab found some facial recognition systems misidentify darker-skinned individuals at higher rates.
- Healthcare Inequality: Diagnostic tools often lack data from specific demographics, leading to less accurate outcomes for minorities.
These examples show the need for proactive steps to reduce bias in AI.
Pro Tip: Ensure Transparency- AI decision-making should be transparent to build user trust.
Quick Guide to Reduce AI Bias
Step 1: Understanding the Sources of Bias
AI bias comes from various sources:
- Data Bias: Training data may reflect societal inequalities, causing AI to repeat discrimination.
- Algorithmic Bias: Some AI models might unintentionally favour specific attributes over others.
- Human Bias: Developers’ biases can affect system design and decision-making.
Recognising these sources is the first step to addressing bias in AI.
Step 2: Implementing Diverse and Representative Data
Diverse data is key to reducing bias in AI.
Strategies include:
- Collecting data from various sources for better demographic representation.
- Using data augmentation to balance underrepresented groups.
- Regularly updating datasets to reflect changing societal norms.
Step 3: Regularly Auditing and Testing AI Systems
Continuous auditing of AI is crucial to identify and reduce bias.
Best practices include:
- Using bias detection frameworks to check algorithmic fairness.
- Conducting third-party audits for impartiality.
- Running tests with real-world scenarios to spot unintended bias.
Step 4: Incorporating Ethical Guidelines in AI Development
Ethical considerations should be part of AI development.
Organisations should:
- Form AI ethics committees to oversee fairness guidelines.
- Create transparent decision-making models.
- Develop metrics to assess AI performance across diverse groups.
Important Tip: Adopt Inclusive AI Development- Encourage diverse teams to reduce unintentional biases.
Common Mistakes and Misconceptions
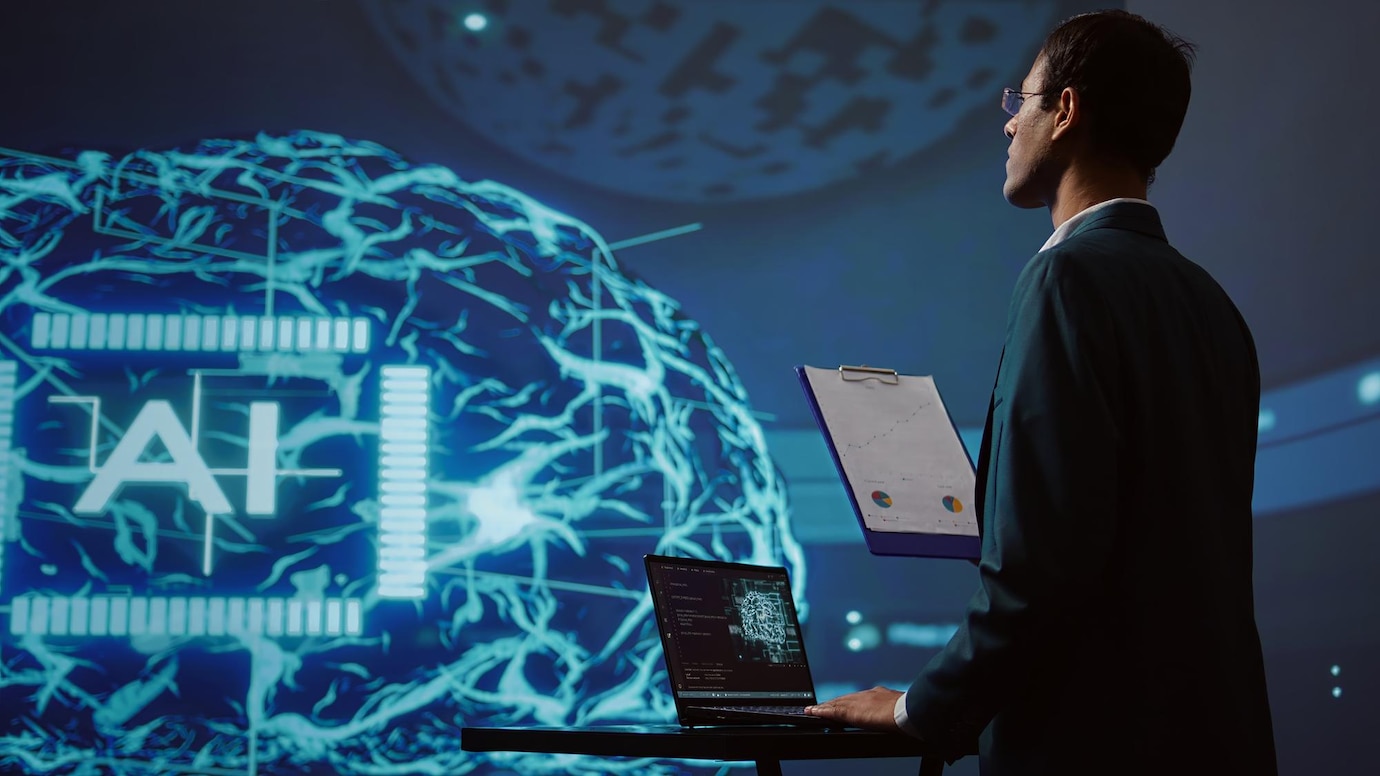
- Assuming AI is Inherently Unbiased: Algorithms reflect biases in their training data.
- Neglecting Human Oversight: AI should support human decision-making, not replace it.
- Focusing Solely on Technical Fixes: Tackling AI bias also means addressing broader societal issues.
Advanced Insights: The Future of AI Fairness
1. Unique Industry Perspectives
Top tech companies are tackling AI bias:
- Google, Microsoft, and IBM have launched initiatives for AI fairness, offering open-source tools and responsible frameworks.
- Explainable AI (XAI) is gaining popularity, helping developers understand and address biases.
2. Emerging Bias Mitigation Techniques
New methods are being developed to combat AI bias:
- Adversarial Debiasing: This technique adjusts training to counteract biases.
- Re-weighting Methods: These change dataset importance to improve fairness.
- Federated Learning: This approach decentralises training across diverse data sources, enhancing fairness while protecting privacy.
3. Legal and Policy Considerations
Regulatory bodies are increasingly focusing on AI fairness:
- The European Union’s AI Act aims to set fairness standards for AI use.
- The U.S. Algorithmic Accountability Act proposes regulations on AI bias in critical decisions.
- Global AI Ethics Frameworks are being discussed for standardised responsible AI guidelines.
The path to unbiased AI has just begun. How will you ensure fairness in AI? Let us know your thoughts, and join the conversation about responsible AI development.
Secret Tip: AI models must be refined to lessen emerging biases.
FAQs
1. Why does AI bias happen?
AI bias results from biased training data, flawed algorithm design, and human biases in development.
2. Can AI ever be truly unbiased?
While eliminating bias is tough, fairness strategies can significantly reduce disparities.
3. How can businesses ensure their AI systems are fair?
Businesses should conduct audits, use diverse datasets, and follow ethical AI guidelines.
4. What role do policymakers play in AI fairness?
Governments create legal frameworks to ensure AI transparency, accountability, and fairness.
5. Are there tools available to detect AI bias?
Tools like IBM’s AI Fairness 360, Google’s What-If Tool and Microsoft’s Fairlearn can help detect and reduce AI bias.
Striving for Fair and Unbiased AI
So, learn to deal with AI bias. It’s a little harder, but you have to. 343 Morning Diff—Data Pollution, AI Building Blocks, Transformer ModelsThe fate of a system depends upon its age.
Collaborative efforts are the key to achieving global fairness in AI across industries, governments, and researchers. AI should benefit all and enhance good social norms rather than reproduce inequalities.